Claude 3.5 Major Upgrade: Large Language Models Now Control Computers Like Humans, Surpassing OpenAI
4102 words (17 minutes)
|AI score: 94 ๐๐๐๐๐

Anthropic recently unveiled a major update to its Claude 3.5 model, introducing the enhanced Claude 3.5 Sonnet and a new model, Claude 3.5 Haiku. Claude 3.5 Sonnet showcases notable advancements in coding abilities and multimodal interaction, particularly with its groundbreaking 'Computer Usage' feature. This feature allows the model to control computers like humans, performing actions such as moving the cursor, clicking, and typing. Currently in public beta testing, this feature primarily targets developers to gather feedback and refine its functionality. While still under development and subject to limitations, the 'Computer Usage' feature holds immense potential, unlocking applications currently beyond the reach of conventional AI assistants. Furthermore, Claude 3.5 Sonnet surpasses OpenAI's o1-mini model in performance and has excelled in various industry benchmarks. Anthropic emphasizes its commitment to security throughout the development process, ensuring that new features operate within established safety standards. With ongoing technological advancements, Claude 3.5 Sonnet is poised to find widespread applications in diverse fields, including software development, automation tasks, and personalized experiences.
Diffusers welcomes Stable Diffusion 3.5 Large
Hugging Face Blog|huggingface.co
1018 words (5 minutes)
|AI score: 94 ๐๐๐๐๐

The article from the Hugging Face Blog announces the release of Stable Diffusion 3.5 Large, an improved version of the previous Stable Diffusion 3 model. The new model is available on the Hugging Face Hub and can be utilized with the Diffusers library. The release includes two checkpoints: a large 8B parameter model and a large 8B timestep-distilled model, which enables few-step inference. The article focuses on the architectural changes in Stable Diffusion 3.5 Large, such as the introduction of QK normalization and dual attention layers, which are standard practices for training large transformer models. Detailed instructions are provided for using Stable Diffusion 3.5 with Diffusers, including installation, model loading, and inference. The article also covers the use of the timestep-distilled model for faster image generation and the application of quantization techniques to optimize memory usage. Additionally, it discusses the training of LoRAs (Low-Rank Adaptation) with quantization and the use of single-file loading for the Stable Diffusion 3.5 Transformer.
Zhipu Open-Sources New Generation Text-to-Image Model CogView3-Plus
738 words (3 minutes)
|AI score: 91 ๐๐๐๐๐
Zhipu announced the open-sourcing of its new generation text-to-image model, CogView3-Plus, on its official WeChat public account. This model builds upon CogView3 with numerous optimizations and upgrades. CogView3 is a text-to-image model based on cascaded diffusion, consisting of three stages: first generating a 512x512 low-resolution image, then generating a 1024x1024 image through a conditional diffusion process, and finally generating a 2048ร2048 high-resolution image. In human evaluation, CogView3 outperformed SDXL, the currently most advanced open-source text-to-image diffusion model, by 77.0%, while requiring only about 1/10 of SDXL's inference time. CogView-3-Plus further introduces the DiT framework, employing Zero-SNR diffusion noise scheduling and text-image joint attention mechanisms, effectively reducing training and inference costs. The model supports flexible generation of resolutions within the 512 to 2048 pixel range and has performed excellently in various evaluations, on par with the most leading text-to-image models. Additionally, the CogView3-Plus series models have been integrated into the Zhipu Qingyan platform, where users can experience its image generation and editing functions. Zhipu has also open-sourced the CogView3-Plus-3B model and plans to build fine-tuning solutions and adapt ControlNet on the Diffusers framework.
GLM-4-Voice: Zhipu AI's Open-Source 'Her' is Here!
้ญๆญModelScope็คพๅบ|mp.weixin.qq.com
1546 words (7 minutes)
|AI score: 92 ๐๐๐๐๐
Zhipu AI recently released GLM-4-Voice, an open-source end-to-end speech model capable of directly understanding and generating Chinese and English speech, enabling real-time dialogue. GLM-4-Voice comprises three key components: GLM-4-Voice-Tokenizer, GLM-4-Voice-Decoder, and GLM-4-Voice-9B. GLM-4-Voice-Tokenizer transforms continuous speech input into discrete tokens by incorporating Vector Quantization into the Whisper Encoder and undergoing supervised training on Automatic Speech Recognition (ASR) data. GLM-4-Voice-Decoder, trained using the CosyVoice Flow Matching model structure, supports streaming inference, converting discrete speech tokens into continuous speech output. GLM-4-Voice-9B, built upon GLM-4-9B, has been pre-trained and aligned with the speech modality, enabling it to understand and generate discrete speech tokens. The research team developed a streaming architecture that facilitates high-quality speech dialogue, allowing the model to adapt its voice characteristics based on user commands while maintaining low latency.
The Evolution of the OpenAI o1 Model
ๅคงๆทๅฎๆๆฏ|mp.weixin.qq.com
15785 words (64 minutes)
|AI score: 93 ๐๐๐๐๐
This article delves into the development and performance of the OpenAI o1 Model, focusing on its remarkable advancements in reasoning ability. It begins by drawing a parallel from the science fiction novel 'The Last Question,' introducing the long-term thinking mode of the artificial intelligence AC. This contrasts with human thinking systems, System 1 and System 2, highlighting the hallucination issues faced by large language models (LLMs) when tackling complex problems, especially in mathematical and logical inference. The article then explains how the OpenAI o1 Model enhances its reasoning capabilities through the Chain of Thought (CoT) method, demonstrating its exceptional performance in the STEM field. The o1 Model surpasses human expert levels in various benchmark tests, particularly in reasoning and multimodal tasks. However, it struggles with text generation and instruction-following tasks. OpenAI has released two versions of o1, prioritizing reasoning ability and processing speed, respectively. The article further explores how the o1 Model incorporates AlphaGo's reinforcement learning approach during training, including Self-Play and Monte Carlo Tree Search (MCTS), along with optimization methods for reasoning generation and reward models. Finally, the article experimentally validates that the Process-supervised Reward Model (PRM) outperforms the Outcome-supervised Reward Model (ORM) in complex reasoning tasks. This is attributed to PRM's ability to provide more precise and frequent error feedback, leading to more effective learning. The article also critiques previous work by Google Deepmind.
Mysterious 'Red Panda' Text-to-Image Model Takes the Lead: Outperforming Flux and Midjourney
1996 words (8 minutes)
|AI score: 90 ๐๐๐๐
A mysterious text-to-image model dubbed 'Red Panda' has emerged as a frontrunner in the field, achieving an ELO ranking over 100 points higher than Flux 1.1 Pro, boasting a 79% win rate, and generating an image every 7 seconds. This model has consistently outperformed established leaders like Flux and Midjourney in text-to-image competitions. The article delves into the impressive capabilities of 'Red Panda', highlighting its performance through comparisons with other models. Despite its remarkable success, the model's origins and developer remain a mystery, fueling speculation and debate. Theories range from Midjourney V7 and OpenAI's DALL-E 4 to a new model from Mistral AI or even a creation from a Chinese manufacturer. Intriguingly, the model's name and logo exhibit a strong Chinese aesthetic, further fueling speculation about its potential Chinese origin. The article also mentions a software engineer whose profile picture name bears a resemblance to the model's name, leading to online speculation about his involvement in its development. The article concludes by encouraging readers to explore 'Red Panda' and speculate on its true identity.
A brief summary of language model finetuning
Stack Overflow Blog|stackoverflow.blog
1345 words (6 minutes)
|AI score: 91 ๐๐๐๐๐
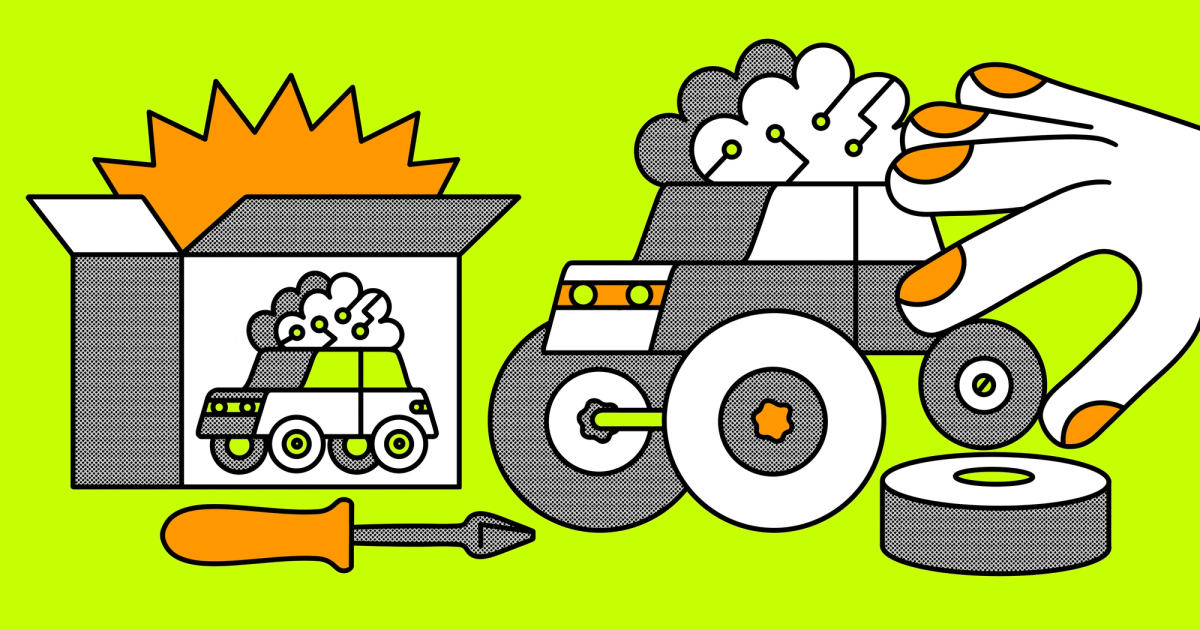
The article delves into the intricacies of fine-tuning techniques for large language models (LLMs), emphasizing the distinction between knowledge injection and alignment. Fine-tuning, which involves further training a pre-trained model, encompasses various methods such as continued pretraining, instruction tuning, supervised fine-tuning (SFT), and reinforcement learning from human feedback (RLHF) or direct preference optimization (DPO). The primary goals of these techniques are to inject new knowledge into the model and to align the model's output style or format with specific requirements. The article highlights the effectiveness of large-scale instruction tuning, exemplified by models like FLAN, which use massive datasets to efficiently solve a wide range of downstream tasks. It also discusses the shift in focus towards alignment after the introduction of ChatGPT, noting that alignment can be achieved with smaller, high-quality datasets, as demonstrated by LIMA. The article further explores the phenomenon of imitating proprietary LLMs, such as GPT-3.5/4, through fine-tuning on small synthetic datasets. While these imitation models perform well on limited benchmarks, they fall short on more extensive evaluations, indicating that fine-tuning can teach style and format but not the extensive knowledge base of more powerful models. The article concludes by summarizing key takeaways, including the importance of understanding the goal of fine-tuning (alignment vs. knowledge injection) and the need for comprehensive benchmarks to assess the effectiveness of fine-tuning. It also mentions ongoing research that continues to explore the boundaries between pretraining and fine-tuning, particularly in understanding when an LLM starts learning new knowledge versus just style or alignment.
Pushing the frontiers of audio generation
Google DeepMind Blog|deepmind.google
976 words (4 minutes)
|AI score: 91 ๐๐๐๐๐
The article from Google DeepMind discusses the latest advancements in audio generation technology, focusing on creating more natural and engaging interactions with digital assistants and AI tools. Key developments include models like SoundStorm and AudioLM, which generate high-quality, natural speech from various inputs. These technologies power several Google products, such as Gemini Live and YouTube's auto dubbing. Two new features, NotebookLM Audio Overviews and Illuminate, make complex content more accessible through AI-generated dialogue. The latest model can produce 2 minutes of dialogue in under 3 seconds on a TPU v5e chip, with improved naturalness and acoustic quality. Future directions include enhancing expressivity and exploring integration with video.
Open-Source Real-Time Dialogue with Customizable Digital Humans: Voice Input and Fast Response
3997 words (16 minutes)
|AI score: 92 ๐๐๐๐๐
This article introduces an open-source demo for real-time dialogue with digital humans, developed by Alibaba's ModelScope Community. The demo allows users to customize the digital human's appearance and provides voice input and real-time conversation capabilities, with an initial response time as low as 3 seconds. The project utilizes a modular design, enabling quick replacement of individual modules, making it suitable for applications such as live streaming, news broadcasting, and chat assistants. Key technical modules include Automatic Speech Recognition (ASR), Large Language Model (LLM), Text-to-Speech (TTS), and Voice Generation (THG), leveraging advanced open-source technologies like FunASR, Tongyi Qianwen, GPT-SoVITS, and MuseTalk. Additionally, the project employs Gradio 5 to achieve streaming video output, facilitating deployment and rapid development of interactive digital human applications. Future optimization plans include link optimization, end-to-end voice chat, and improvements in streaming video playback.
Stanford's Open-Source Academic Research Tool STORM Evolves: AI Agents Collaborate in Roundtable Discussions
1581 words (7 minutes)
|AI score: 90 ๐๐๐๐

Stanford University launched the open-source tool STORM in April, leveraging large language models (LLMs) to assist in writing Wikipedia-like articles and facilitating the rapid generation of detailed research papers. Recently, the Stanford team introduced the upgraded version, Co-STORM, which incorporates a collaborative dialogue mechanism and turn-taking strategy to enable AI agents to engage in roundtable discussions. Co-STORM comprises three types of agents: LLM experts, moderators, and human users. These agents dynamically update a knowledge graph and generate questions or answers, significantly enhancing the quality and efficiency of academic research. Evaluation results demonstrate that Co-STORM outperforms baseline systems in both report quality and dialogue quality, particularly in terms of depth and novelty.
Using Agents as Judges: AI Agents Self-Evaluate, Cost Plummets by 97%
2496 words (10 minutes)
|AI score: 92 ๐๐๐๐๐
A recent study from Meta and KAUST teams introduces the 'Agent-as-a-Judge' framework, addressing the challenge of evaluating AI agent decision paths. Traditional evaluation methods either focus solely on results or require extensive human intervention. This new framework allows agents to self-evaluate, reducing costs and time by 97% and providing rich intermediate feedback. This framework is an organic extension of 'LLM-as-a-Judge,' incorporating agent characteristics to provide intermediate feedback throughout the task resolution process. The research team also introduced the DevAI benchmark, containing 55 real AI development tasks with detailed manual annotations, to validate the effectiveness of the new framework. Experimental results show that the new framework outperforms traditional 'LLM-as-a-Judge' frameworks in evaluating agent systems, especially in the context of task dependencies. Additionally, the article discusses the shortcomings of current code generation benchmark tests and introduces the DevAI dataset, aimed at addressing issues in current benchmarks.
Digital Human Creation Without Training: ByteDance PersonaTalk Video Lip-Sync Editing Surpasses State-of-the-Art
2462 words (10 minutes)
|AI score: 91 ๐๐๐๐๐
In the wave of AI-Generated Content (AIGC), video lip-sync editing technology has become a crucial tool for personalized and intelligent video content. ByteDance's PersonaTalk technology, recently selected for the SIGGRAPH Asia 2024-Conference Track, achieves high-quality video lip-sync editing through an attention-based two-stage framework, enabling digital human creation without training. PersonaTalk combines the advantages of customized training and zero-shot solutions, generating high-quality videos through a style-aware animation generation module and a dual-branch parallel attention module. Experimental results demonstrate that PersonaTalk outperforms other state-of-the-art (SOTA) solutions in lip synchronization, visual quality, and personalization feature retention, even surpassing the latest customized training solutions in academia without additional training or fine-tuning. This technology has broad application prospects, including video translation, virtual teachers, AIGC creation, and more, providing new ideas for innovation across multiple fields.
A Comprehensive Guide to Large Models
ไบบไบบ้ฝๆฏไบงๅ็ป็|woshipm.com
23459 words (94 minutes)
|AI score: 92 ๐๐๐๐๐
This article offers a detailed explanation of the fundamental concepts, key technologies, and application scenarios of large models, aiming to provide AI novices and product managers with a comprehensive understanding. It begins by defining large models, such as GPT-4.0, and explaining their use of the Transformer architecture and their ability to generate text. The article then delves into the key technologies behind large models, including pre-training, model fine-tuning, prompt engineering, model distillation (a technique for transferring knowledge from a larger model to a smaller one), and model pruning (reducing model complexity). It also clarifies the relationships between AI, machine learning, deep learning, and NLP. Furthermore, the article introduces the main applications of large models, such as text generation, dialogue systems, and question-answering systems, and discusses the advantages of the MoE Architecture. The article further elaborates on the principles, classification, core technologies, and development steps of text generation by large models, covering the entire process from text generation to model optimization. Finally, the article examines the key elements of training and fine-tuning large models, including data requirements, training costs, fine-tuning methods, and the main factors and evaluation dimensions affecting large model performance. It also explores the limitations of large models, such as 'hallucination' (generating seemingly plausible but inaccurate information), 'amnesia' (forgetting information), and 'inappropriate content generation', and discusses solutions like prompt engineering, Retrieval-Augmented Generation (RAG), and fine-tuning.
Cursor: How to Build Best Practices for AI Coding?
ๆตทๅค็ฌ่งๅ ฝ|mp.weixin.qq.com
24075 words (97 minutes)
|AI score: 92 ๐๐๐๐๐
Cursor is an integrated development environment (IDE) built upon large language models (LLMs), with a focus on the AI Coding field. This article delves into the details of Cursor's product experience, model training, data security, and explores the future trajectory of AI coding and AI Agents. Cursor leverages the Claude Sonnet 3.5 model to enhance coding capabilities and continues to invest in AI Coding UI/UX. The team is experimenting with the Shadow Space product concept, where coding tasks are handled in hidden windows running in the background. Future programming will be a harmonious blend of natural language and code, with AI reshaping the programming experience, boosting efficiency while preserving programmers' creativity and control. Furthermore, Cursor has implemented a more intelligent code editing experience through the Tab key, enabling the model to automatically identify and suggest the next location requiring editing, reducing the user's operational burden. Cursor also optimizes the response speed of AI Coding products through speculative editing, cache warmup, and advanced cache mechanisms. By training models with Reinforcement Learning (RL), it predicts user preferences and optimizes suggestion generation, enhancing user experience and model performance.
Bringing developer choice to Copilot with Anthropicโs Claude 3.5 Sonnet, Googleโs Gemini 1.5 Pro, and OpenAIโs o1-preview
583 words (3 minutes)
|AI score: 91 ๐๐๐๐๐
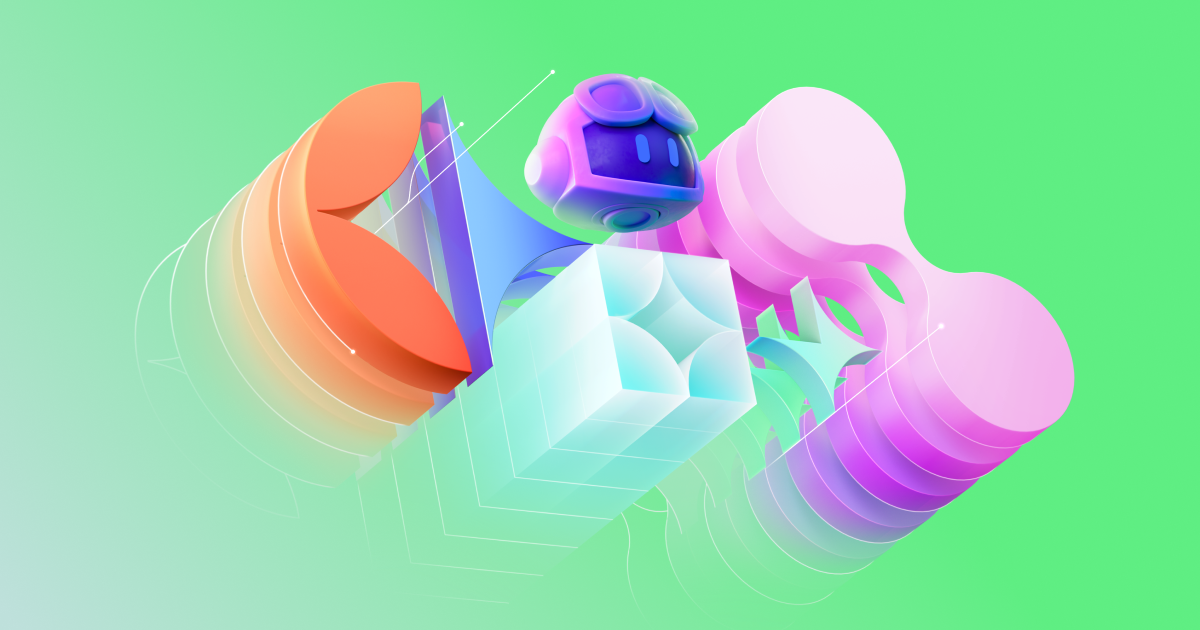
GitHub Copilot, an AI-powered coding assistant, has been evolving by integrating various large language models (LLMs) to enhance its functionality. Initially launched with OpenAI's Codex, a variant of GPT-3 fine-tuned for coding, Copilot has since expanded its capabilities by incorporating GPT-3.5 and GPT-4. The recent update introduces a multi-model choice feature, allowing developers to select from Anthropic's Claude 3.5 Sonnet, Google's Gemini 1.5 Pro, and OpenAI's o1-preview and o1-mini. This move underscores GitHub's commitment to providing developers with the flexibility to choose the model that best suits their needs, whether for coding, debugging, or optimizing code. The new models bring distinct advantages: Claude 3.5 Sonnet excels in handling complex, multi-step coding tasks; Gemini 1.5 Pro offers a multi-modal approach with a large context window; and OpenAI's o1-preview and o1-mini enhance reasoning capabilities for better understanding code constraints and edge cases. This multi-model choice is being rolled out across various Copilot features, including Copilot Chat, Workspace, multi-file editing, and more. Additionally, GitHub introduced GitHub Spark, an AI-native tool for building applications using natural language, showcasing the platform's broader vision to support 1 billion developers. GitHub Spark allows users to create micro apps with AI features and external data integration without managing cloud resources, leveraging a creativity feedback loop for iterative development.
How to Write Effective Prompts?
190 words (1 minutes)
|AI score: 91 ๐๐๐๐๐
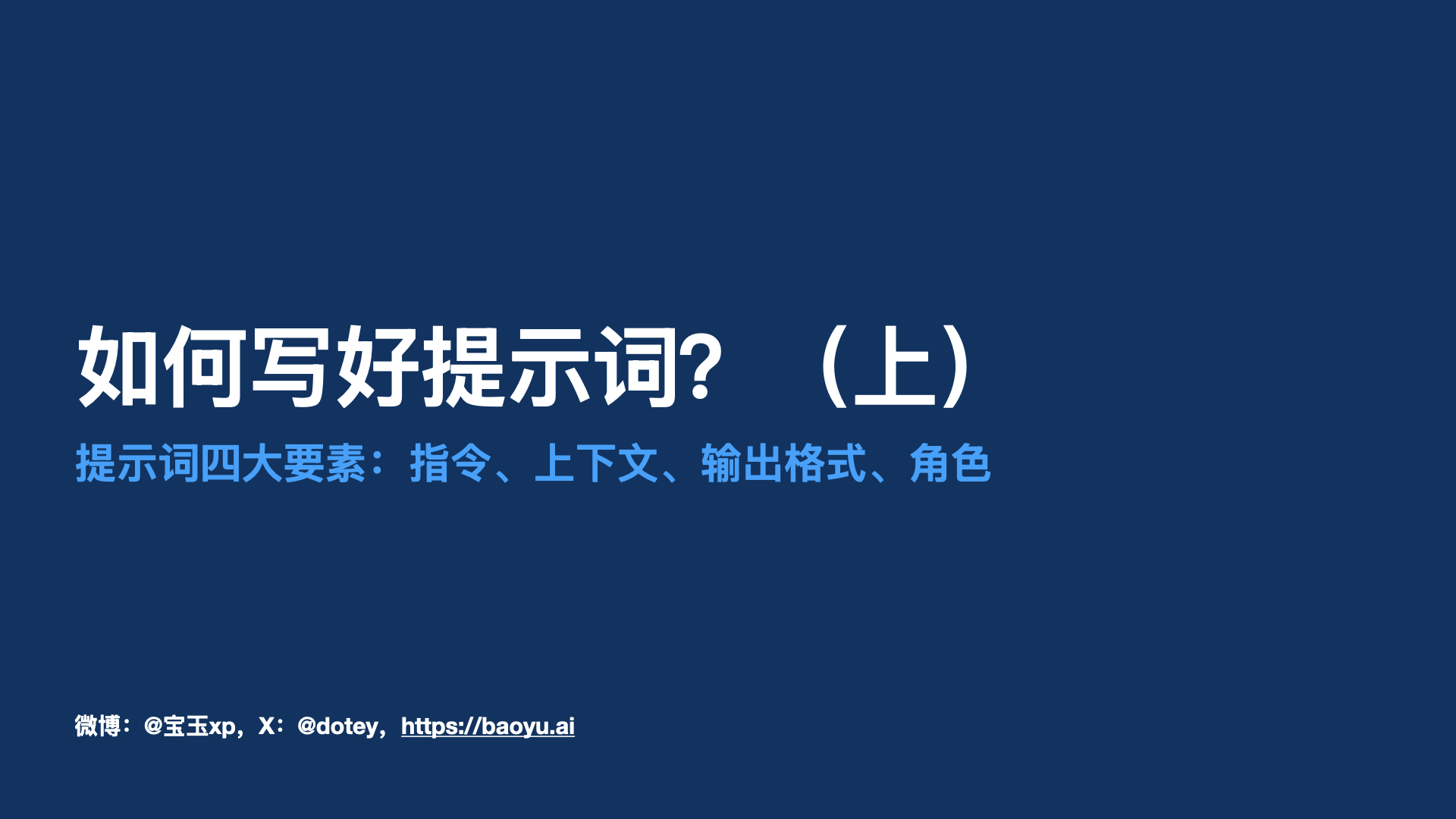
Baoyu shares this article, detailing methods and techniques for writing effective prompts. The author created a 2-hour instructional video and prepared 110 pages of slides to thoroughly explain various aspects of prompt engineering. While the author modestly claims the video quality is not high, the content is meticulously prepared and offers valuable insights. The article also provides links to the video, available on YouTube and Bilibili (Chinese video platform), as well as download links for the slides, facilitating in-depth learning and reference for readers.
Beyond CLIP: How Jina-CLIP Advances Multimodal Search
2427 words (10 minutes)
|AI score: 91 ๐๐๐๐๐

The article introduces Jina-CLIP v1, a new multimodal embedding model developed by Jina AI, which enhances the capabilities of multimodal search by combining text and image data. The model addresses the limitations of OpenAI's CLIP, particularly in handling longer texts and complex textual relationships. Jina-CLIP v1 uses a smarter text understanding model (JinaBERT) and is trained to match text to both images and other texts, simplifying the search process and reducing the need for separate models for different modalities. The article details two key experiments demonstrating the model's effectiveness: improving search results by combining text and image search, and using images to diversify search results. The experiments, conducted using the Fashion200k dataset, show that averaging text and image embeddings yields better retrieval results, maintaining search quality while incorporating visual cues for more diverse and well-rounded results. Additionally, using image embeddings as a 'visual reranker' enhances result variety while maintaining relevance. The article concludes by highlighting the potential applications of Jina-CLIP v1 in various fields, including e-commerce, media asset management, and visual content curation, and mentions the upcoming multilingual support in Jina-CLIP v2.
Jina Classifier API for High Performance Zero-Shot and Few-Shot Classification
2579 words (11 minutes)
|AI score: 91 ๐๐๐๐๐
The article introduces Jina AI's new Classifier API, designed to support high-performance zero-shot and few-shot classification tasks. Built on advanced embedding models like jina-embeddings-v3
and jina-clip-v1
, the API leverages online learning to adapt to new data in real-time, allowing users to start with zero-shot capabilities and incrementally update with new examples. The API supports both text and image classification, making it versatile for various content types, and allows users to publish their classifiers for public use. Detailed examples demonstrate the API's use in LLM query routing, multimodal content categorization, and detecting genuine content from Jina Reader. The article also discusses the importance of semantically meaningful labels in zero-shot classification and the stateless nature of the model. Few-shot classification is highlighted for its ability to adapt to new examples and evolve over time with minimal labeled data. The benchmark analysis shows that both zero-shot and few-shot methods achieve comparable accuracy around the 400-sample mark, with few-shot maintaining a slight edge when some training data is available.
How We Built a Content Recommendation System With Pgai and Pgvectorscale
4264 words (18 minutes)
|AI score: 91 ๐๐๐๐๐

The article from Timescale Blog details the development of a content recommendation system by Pondhouse Data, focusing on the use of pgai and pgvectorscale extensions within PostgreSQL. The project aims to simplify AI application development, particularly for small teams, by reducing complexity and leveraging large language models (LLMs) without extensive infrastructure setup. The system is designed to support multi-tenant applications, crucial for industries with high data privacy concerns. pgai, a PostgreSQL extension, allows direct interaction with LLM APIs through database queries, enabling tasks like tagging, content moderation, summarization, and creating vector embeddings. pgvectorscale, another PostgreSQL extension, addresses scalability issues with vector search by introducing a disk-based streaming index, enhancing performance and flexibility. The article provides a step-by-step guide to building an SEO-focused content recommendation system, emphasizing the importance of internal linking for SEO and user engagement. It outlines the process from summarizing content and creating embeddings to searching for similar content and suggesting inline links. The guide includes practical steps for installation and setup, using pre-built TimescaleDB images and OpenAI API integration. Overall, the article demonstrates the efficiency and simplicity of using pgai and pgvectorscale for AI-driven content recommendation systems, highlighting their potential to revolutionize content management and SEO strategies.
Expert Support case study: Bolstering a RAG app with LLM-as-a-Judge
Hugging Face Blog|huggingface.co
2061 words (9 minutes)
|AI score: 91 ๐๐๐๐๐
The article presents a case study of Farmer.chat, an AI-powered chatbot developed by Digital Green in collaboration with CGIAR and mentored by Hugging Face through their Expert Support program. The chatbot aims to provide personalized and reliable agricultural advice to smallholder farmers and extension workers, leveraging a vast knowledge base of agricultural research papers. The system architecture includes a knowledge base, a RAG pipeline, and a user-facing agent powered by GPT-4. The article highlights the challenges of creating a chatbot that can cater to diverse languages, geographies, crops, and use cases, emphasizing the importance of context-specific and accurate information dissemination. To evaluate the performance of the RAG pipeline, the team introduced an LLM-as-a-judge system, which assesses the clarity of user prompts, the type of questions asked, the percentage of answered queries, and the accuracy of the RAG responses. This method allows for a more nuanced understanding of the chatbot's effectiveness and user experience. The article also discusses the benchmarking of different LLMs (GPT-4-Turbo, Llama-3-70B, Gemini-1.5-Pro, and Gemini-1.5-Flash) for their faithfulness and relevance in answering agricultural queries, ultimately selecting Gemini-1.5-Flash for its superior trade-off between low unanswered questions and high faithfulness. The conclusion emphasizes the benefits of using LLMs as judges for improving user experience, optimizing the knowledge base, and selecting the right LLMs for specific tasks. Farmer.chat has served over 20k farmers, answered more than 340k questions, and supports multiple languages and crops.
Building Vectorize, a distributed vector database, on Cloudflareโs Developer Platform
The Cloudflare Blog|blog.cloudflare.com
4081 words (17 minutes)
|AI score: 93 ๐๐๐๐๐
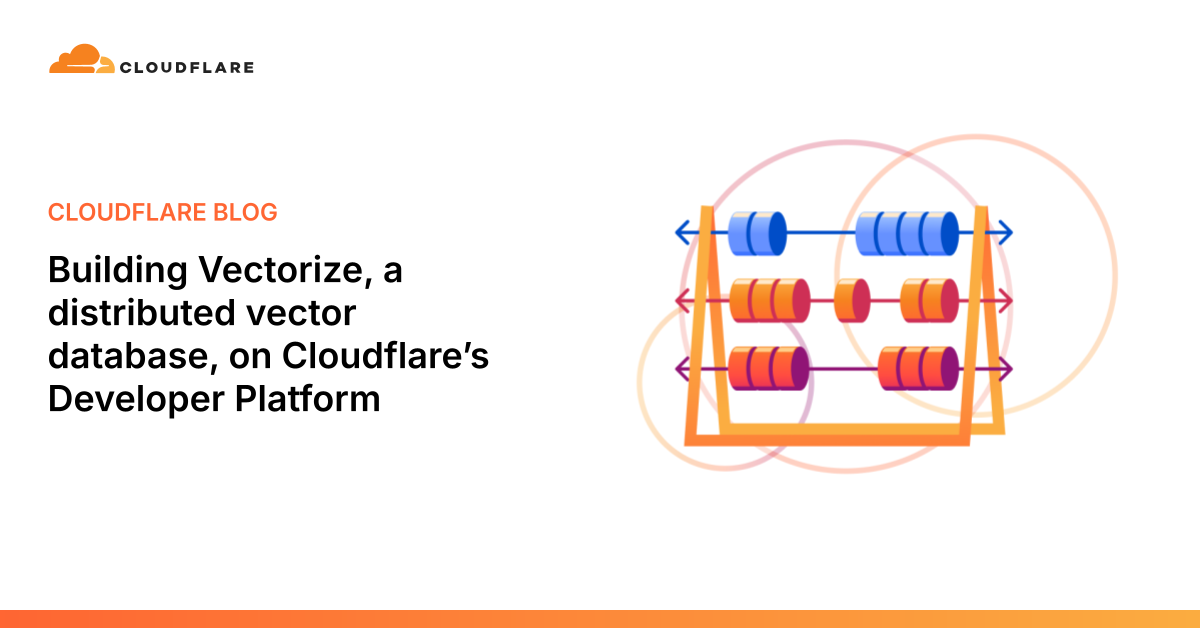
Cloudflare has developed Vectorize, a distributed vector database on its Developer Platform, designed to support full-stack AI-powered applications through Cloudflare Workers. Vectorize enhances the querying of embeddings, which are representations of data like text and images, making them faster, easier, and more cost-effective. The article delves into the architecture of Vectorize, explaining how it utilizes Cloudflare's global network, R2 object storage, and caching to optimize I/O operations. It also discusses advanced techniques like IVF for search space pruning and PQ for vector compression, ensuring efficient similarity search even with large datasets. Additionally, the article covers eventual consistency and snapshot versioning to maintain data integrity during concurrent writes, and the implementation of a WAL for coordinating distributed writes and ensuring atomic updates.
How to Choose a Vector Database
2198 words (9 minutes)
|AI score: 92 ๐๐๐๐๐
The article begins by highlighting the transformative impact of large language models (LLMs) and generative AI on data management, particularly the rise of vector databases as crucial components in modern AI and machine learning applications. It explains the significance of vector embeddings in representing semantic meaning of various data types, enabling efficient and meaningful data retrieval. The article then delves into the diverse applications of vector databases, such as RAG for chatbots, semantic search for product catalogs, recommendation systems, and more. The core of the article focuses on key evaluation criteria for choosing a vector database, including query rate, partition-ability, secondary filtering needs, system of record considerations, data changes and synchronization, and handling structured data. It discusses the trade-offs between serverless and dedicated vector databases, emphasizing their suitability based on query rates and application requirements. The article also contrasts general-purpose and specialized vector databases, advocating for the versatility of general-purpose databases like PostgreSQL with extensions like pgvector. Additional considerations such as performance, security and reliability, developer experience, and observability are discussed in detail. The article concludes by emphasizing the importance of understanding application needs and system requirements to choose the best vector database, recommending Timescale Cloud for its comprehensive capabilities in handling metadata, vector embeddings, and time-series data.
5 Chunking Strategies for RAG [Translated]
1331 words (6 minutes)
|AI score: 90 ๐๐๐๐
![5 Chunking Strategies for RAG [Translated]](https://baoyu.io/uploads/2024-10-22/1.webp)
This article delves into how different chunking strategies can be employed to handle large documents in RAG applications, improving retrieval efficiency and the quality of generated responses. RAG, a method combining retrieval and generation techniques, stores additional information as vectors and matches them with incoming queries, ultimately passing the most relevant information to the large language model (LLM). As documents can be extensive, the chunking operation becomes crucial to ensure text fits within the input size of the embedding model, enhancing the efficiency and accuracy of the retrieval step. The article outlines five chunking strategies: fixed-size chunking, semantic chunking, recursive chunking, document structure-based chunking, and LLM-based chunking. Each strategy possesses its advantages and disadvantages, and the final choice depends on the nature of the content, the capabilities of the embedding model, and computational resources.
Exploration and Application of Large Models in Huawei's Recommendation Scenarios
8600 words (35 minutes)
|AI score: 93 ๐๐๐๐๐
Huawei's exploration and application of large models in recommendation scenarios have addressed the limitations of traditional recommendation systems by incorporating open-domain knowledge and collaborative information, significantly enhancing recommendation effectiveness. The article details various applications of large models in recommendation systems, including feature engineering, encoder enhancement, direct scoring and ranking, and conversational interaction. Huawei has also explored personalized retrieval and fuzzy verification methods, significantly improving the acceleration effect and long sequence understanding capability of large models. By using personalized parameter fine-tuning methods, Huawei has successfully injected knowledge from the recommendation system domain into large models, enhancing the model's prediction effect and training efficiency, while also addressing the issue of high inference latency.
Exploring Methods to Restrict JSON Format Output Throughout the Entire LLM Inference Stage
้ฟ้ไบๅผๅ่ |mp.weixin.qq.com
3651 words (15 minutes)
|AI score: 91 ๐๐๐๐๐
This article explores how to ensure that large language models (LLMs) output structured JSON formats during the inference process, emphasizing its role in improving data processing automation and system interoperability. The article analyzes the reasons why LLMs struggle to strictly output JSON formats during inference, pointing out that the prediction and sampling mechanisms of LLMs determine that they cannot output JSON 100% as required. To address this issue, the article proposes optimization strategies for three stages: 1. Pre-inference (Prompt Engineering): By designing carefully crafted prompts, the probability of JSON output is increased. 2. Mid-inference (Dynamic Constrained Decoding): During the inference process, dynamic constrained decoding technology ensures that LLMs strictly follow the predefined JSON schema for output, achieving 100% JSON format output. 3. Post-inference (Post-Processing): After model output, post-processing techniques (such as the JSON Repair library) are used to correct the JSON structure, further improving the accuracy of JSON output. The article also introduces OpenAI's Structured Outputs method and details the implementation process of dynamic constrained decoding, including local model deployment and using regular expressions to restrict output formats. Finally, the article summarizes the advantages and disadvantages of the three methods and looks forward to their application in more scenarios in the future.
LlamaIndex Newsletter 2024-10-29
563 words (3 minutes)
|AI score: 91 ๐๐๐๐๐
The LlamaIndex newsletter for October 29, 2024, offers a comprehensive update on the latest developments and use cases of LlamaIndex and LlamaCloud. Key highlights include: 1) Knowledge Management for RFP Response Generation, demonstrating how LlamaCloud indexes unstructured data to support complex agent workflows for generating RFP reports; 2) A step-by-step guide to building a customer service system using LlamaIndex, showcasing the platform's capabilities in multi-agent orchestration and human-in-the-loop features; 3) A new video series on advanced knowledge assistants, covering topics from RAG basics to auto-retrieval and corrective RAG techniques using LlamaCloud; 4) The Gift Genie Project, a hackathon project where an agentic system generates and debates gift ideas, highlighting creative uses of AI in decision-making processes. Additionally, the newsletter includes community tutorials, webinars, and recruitment opportunities, emphasizing LlamaIndex's commitment to fostering a vibrant developer community and expanding its team.
Deep Dive into RAG: A Solution for Knowledge-Intensive NLP Tasks
ๅคงๆทๅฎๆๆฏ|mp.weixin.qq.com
5153 words (21 minutes)
|AI score: 91 ๐๐๐๐๐
In the face of increasingly knowledge-intensive tasks, RAG technology significantly enhances the generation capabilities of language models by retrieving relevant information from external memory sources. This article begins by introducing the fundamental concepts and workings of RAG, highlighting how its combination of a retriever and a generator effectively improves the accuracy and relevance of model generation. Subsequently, the article analyzes the application scenarios of RAG, including retrieving external knowledge, retrieving contextual history, retrieving training examples in context, and retrieving tool-related information, demonstrating RAG's advantages in addressing data privacy, real-time data processing, and hallucination issues. However, RAG also presents limitations and challenges, such as reliance on text fragment retrieval, limitations in the retrieval process, and the potential introduction of contradictory documents. The article further explores the technical challenges in the practical implementation of RAG, including latency issues, cost considerations, factual errors and hallucinations, and technical and optimization challenges. Finally, the article summarizes the application value and future development direction of RAG, emphasizing its potential in lowering the barriers to AI applications and improving efficiency.
Alibaba Cloud AI Search RAG Large Model Optimization Practices
7192 words (29 minutes)
|AI score: 92 ๐๐๐๐๐
Ou Mingdong, a senior algorithm expert at Alibaba Cloud, shared Alibaba Cloud's practical experience in optimizing the Retrieval-Augmented Generation (RAG) large model. The article details how Alibaba Cloud enhances the effectiveness and performance of RAG through document structuring, large model fine-tuning, and Agent Technology. RAG technology excels in addressing issues such as large model hallucinations and outdated information, particularly in knowledge base Q&A and web search. Alibaba Cloud decomposes complex problems using Agent Technology to optimize system modules, including the data layer, offline services, and online engines, significantly improving search and answer quality. Additionally, through model fine-tuning and the introduction of Agent planning, Alibaba Cloud has achieved notable results in handling complex problems and reducing hallucination rates. The article also mentions the practical applications of RAG in e-commerce, content, enterprise knowledge base, and educational question search scenarios, showcasing its processing flow and optimization strategies.
Understanding AI from an Architectural Perspective: A Guide for Architects on Machine Learning and Generative Augmentation Techniques
14280 words (58 minutes)
|AI score: 92 ๐๐๐๐๐
This article aims to guide architects in understanding and effectively applying machine learning (ML) and artificial intelligence (AI) technologies, especially generative AI (genAI) and Large Language Models (LLMs). It begins by highlighting the crucial step of defining success criteria before implementing LLMs and introduces prompt engineering and Retrieval-Augmented Generation (RAG) as essential techniques for improving LLM performance. Vector databases enhance LLM's context understanding by enabling efficient nearest neighbor search, allowing them to quickly identify relevant information from large datasets. The article then delves into the fundamental principles of machine learning models, including probability distributions, language models, matrix multiplication in neural networks, and the scale of large language models. The concept and training process of generative AI, along with the role of platforms like Hugging Face, are also explored in detail. Additionally, the article covers the lifecycle of machine learning models, the operation mechanism of autoregressive models, the concept of tokens and their application in models, and the importance of the Transformer architecture in language models. In strategies for integrating LLMs into products, the article analyzes the pros and cons of using commercial LLMs and self-hosted open-source LLMs, as well as how to compare the performance of different LLMs and define success criteria. The application of Retrieval-Augmented Generation (RAG) and fine-tuning techniques in specific domains, and the role of vector databases in nearest neighbor search, are also discussed in detail. Finally, the article explores LLM's application in natural language processing, the differences between AI Copilot and AI Agent, and issues of generalizability and autonomy in artificial intelligence through a dialogue format.
Microsoft Open-Sources OmniParser: Empowering Everyone to Build Computer and Smartphone Control Agents
1845 words (8 minutes)
|AI score: 91 ๐๐๐๐๐
Microsoft has released OmniParser, a groundbreaking open-source tool that leverages large language models to parse user interface (UI) screenshots into structured elements. OmniParser's ability to analyze and understand UI surpasses even GPT-4V, setting a new standard in screen parsing. By parsing UI screenshots, OmniParser identifies interactive icons and deciphers the meaning of various screen elements, accurately linking planned actions to their corresponding areas. This capability opens up a wide range of practical applications, such as parsing web pages and executing specific tasks. OmniParser's effectiveness is further demonstrated by its superior performance in multiple benchmark tests, including ScreenSpot, Mind2Web, AITW, and WindowsAgentArena. The development of OmniParser involved creating a dedicated dataset and fine-tuning detection and description models, enabling it to handle diverse operating systems and application interfaces with robustness. Moreover, OmniParser can be integrated as a plugin for various vision-language models (VLMs), further enhancing AI's ability to control computers. The open-source nature of OmniParser fosters widespread adoption and unlocks its potential for diverse applications.
Introducing AI-driven BigQuery data preparation
Google Cloud Blog|cloud.google.com
763 words (4 minutes)
|AI score: 90 ๐๐๐๐
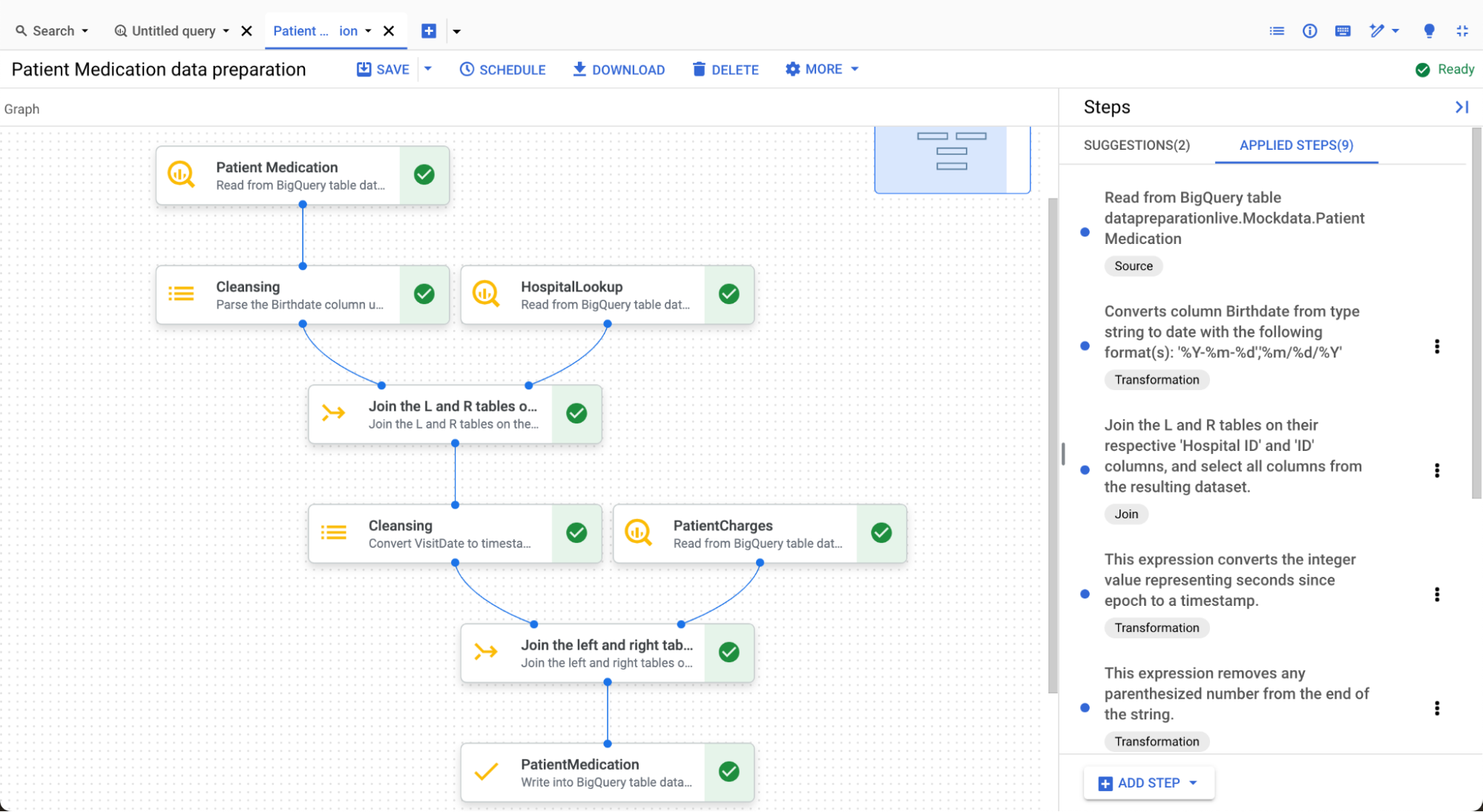
The article introduces an AI-driven data preparation tool within Google's BigQuery, aiming to streamline and simplify the process of transforming raw data into actionable insights. This solution, part of the Gemini in BigQuery ecosystem, leverages AI to provide intelligent suggestions for data cleaning, transformation, and enrichment, thereby reducing manual effort and time spent on data preparation. Key features include AI-powered suggestions, data cleansing and standardization, visual data pipelines, and data pipeline orchestration. The tool integrates with other Google Cloud services like Dataform and Cloud Storage, offering a unified and scalable environment for data management. The article also highlights customer testimonials from companies like GAF, mCloud Technologies, and Public Value Technologies, showcasing the practical benefits and adoption of the tool.
Tongyi's New Product: Code Mode for Non-Programmers
3452 words (14 minutes)
|AI score: 91 ๐๐๐๐๐
Tongyi, under Alibaba, announced a new product called 'Code Mode' on October 24th and opened trial reservations, inviting the first batch of 1024 users to experience it. This mode aims to lower the barrier to application development, especially for non-professional programmers. It offers real-time preview and visual editing for a more intuitive experience. Tongyi Code Mode is based on the Qwen 2.5 large model, capable of generating code in real-time and previewing it on the web, supporting over 40 programming languages, significantly enhancing code generation and reasoning capabilities. This mode not only simplifies the code generation process but also provides intuitive visual results, allowing people without development experience to quickly realize new ideas. The launch of Tongyi Code Mode signals a new round of iteration in AI development, potentially becoming an important direction for future AI development.
ChatGPT Now Offers AI Search, Free to Use
3640 words (15 minutes)
|AI score: 92 ๐๐๐๐๐

OpenAI has announced the launch of ChatGPT search, marking its official transformation into an AI search engine. This update addresses ChatGPT's previous limitations in accessing real-time information, allowing users to quickly find answers through web resource links. Paid subscribers and waitlist users already have access to real-time conversational information, while free users, enterprise users, and educational users will gain access in the coming weeks. The feature is available on ChatGPT's web version, mobile and desktop apps, allowing users to actively trigger web searches or have ChatGPT decide when to use web search results based on their needs. OpenAI emphasizes that ChatGPT search aims to provide better answers by using a more natural conversational questioning method, combining web information for responses, and providing deeper answers based on chat context. To supplement the latest information, OpenAI has partnered with news and data providers and plans to introduce new visual designs for different categories. OpenAI has also stated that there are currently no plans to place ads in ChatGPT, making it a more user-friendly experience compared to traditional search engines. In terms of technical implementation, ChatGPT search is a fine-tuned version of GPT-4o, utilizing content from third-party search providers and partners. OpenAI is actively recruiting Google employees to join its search team and plans to continuously improve the search experience, especially in shopping and travel areas. OpenAI also plans to introduce the new search experience into advanced voice and canvas, and will continue to update the large language model's data to ensure users get the latest progress. The article also mentions OpenAI's AMA Q&A session on Reddit, where Sam Altman and Kevin Weil answered questions about ChatGPT-5, text-to-image models, achieving AGI, open source strategy, model naming, camera mode, image input support, inference cost reduction, best use cases, NSFW content support, GPT product line breakthroughs, o1 full version improvements, and 2025 predictions. Finally, the article mentions that Google almost simultaneously announced its own AI search feature, launching the Grounding feature, allowing Gemini API and Google AI Studio users to obtain real-time information from Google search, signaling the beginning of the AI search war.
Interview with Kyth: How Little Universe CEO Understands AI Podcasts?
7777 words (32 minutes)
|AI score: 90 ๐๐๐๐
This article, through a conversation with Little Universe CEO Kyth, delves into the essential differences between AI podcasts and human podcasts, emphasizing the core value of human emotions and authenticity in podcasts. Kyth believes that while AI can enhance podcast creation, it cannot replace the uniqueness of human hosts and the audience's demand for authenticity. Additionally, the article discusses the development trends of the podcast industry, including how podcasts penetrate different circles, the increase in content supply, podcasts as a new front for brand marketing, and the future commercialization focus of podcasts. Kyth also shares Little Universe's strategies for commercialization and videoization, as well as his views on the long-term trends of the podcast industry. Finally, Kyth emphasizes the unique value of podcasts in emotional connection and user companionship, believing that podcasts can become a 'refuge' and 'corner of peace' for users in times of anxiety and confusion.
LangChain Founder's In-Depth Explanation: A Step-by-Step Guide to Designing Agent User Interaction
9099 words (37 minutes)
|AI score: 91 ๐๐๐๐๐
This article, written by LangChain founder Harrison Chase, explores in detail the definition, design, and user interaction of Agents. The article first defines Agents and emphasizes the importance of Agent characteristics in development, operation, and evaluation. It then analyzes the planning and reasoning capabilities of Agents, pointing out the limitations of current Large Language Models (LLMs) in this area and proposing methods to enhance Agent performance through domain-specific cognitive architectures. Subsequently, the article discusses user interaction patterns in Agent systems, comparing the advantages and disadvantages of streaming chat and non-streaming chat, and looking forward to more possible UX forms in the future. Additionally, the article explores how Agents build user trust when running in the background, introducing emerging user interaction methods such as spreadsheet user experience, generative UI, and collaborative UX. Finally, the article discusses UX design for Agent collaboration with humans, particularly the differences between collaborative UX and environmental UX, emphasizing different needs for concurrency and work presentation methods.
EvenUp, a Legal AI Company Valued at Over $1 Billion, Helps 1,000 Law Firms Recover $1.5 Billion in Compensation
6154 words (25 minutes)
|AI score: 92 ๐๐๐๐๐
EvenUp, a Legal Technology company specializing in using AI to handle personal injury claims, recently secured $135 million in funding, pushing its valuation beyond $10 billion. The company's AI tool, Piaiโข, automates the generation of claim letters and medical timelines for lawyers, significantly improving case processing efficiency and accuracy. EvenUp has partnered with over 1,000 law firms, successfully recovering $1.5 billion in compensation, highlighting the significant commercial value of AI in the legal field. The article also emphasizes the critical role of data in AI implementation, noting that technological barriers are gradually diminishing, and high-quality data sets are becoming the core factor determining the effectiveness of AI applications. Additionally, the article provides links to several related topics, covering AI applications, startups, product managers, and YouTube monetization.
Magic Fill + Image Extension, Ideogram Launches AI Canvas Tool
994 words (4 minutes)
|AI score: 91 ๐๐๐๐๐

Ideogram recently launched its AI Canvas tool, Canvas, showcasing powerful functionality and creative potential in the field of image generation and editing. Canvas's core features include Magic Fill and Image Extension, allowing users to easily perform tasks such as object replacement, text addition, defect repair, and background replacement. Users simply select the unchanged areas and describe their desired content or scene using text. AI will then automatically handle the complex image processing tasks. Additionally, Canvas can seamlessly connect two independent images, creating a naturally harmonious image. It also adds text with consistent style on existing images, addressing a common shortcoming of most image generation applications in handling text within images. The Infinite Canvas feature supports creating infinite zoom animations, further enhancing its creative expression capabilities. While Canvas currently focuses on image generation and lacks some user drawing and element linking features found in mainstream canvas tools, its powerful image processing capabilities have attracted significant attention from users, including OpenAI's founding member Andrej Karpathy. Currently, Canvas's basic functions are free for all users, but advanced features like Magic Fill and Image Extension require a paid subscription.
6 Types of Conversations with Generative AI
ไบบไบบ้ฝๆฏไบงๅ็ป็|woshipm.com
5136 words (21 minutes)
|AI score: 91 ๐๐๐๐๐
This article analyzes 425 interactions with Generative AI dialogue robots like ChatGPT, Bing Chat, and Bard, identifying six common dialogue types: Search Query Dialogue, Guided Dialogue, Investigative Dialogue, Multifaceted Dialogue, Iterative Dialogue, and Precision Targeting Dialogue. Each type has specific usage scenarios and design requirements. The article details the characteristics, user needs, and design suggestions for each type, aiming to help users interact more effectively with AI and provide designers with practical guidelines for optimizing the AI dialogue experience. The article emphasizes that different dialogue types meet different information needs, and there is no optimal dialogue length; the key lies in providing the appropriate amount of information based on user goals. Additionally, the article discusses the impact of dialogue duration, pointing out that the number of dialogue rounds is not directly related to the ease of information acquisition, but depends on the clarity of the initial prompt and the user's information needs.
AI-Driven Design: Exploring the 'Pinch for Summary' Feature
ไบบไบบ้ฝๆฏไบงๅ็ป็|woshipm.com
2982 words (12 minutes)
|AI score: 90 ๐๐๐๐
In the digital age, users demand more immediate, accurate, and personalized information access. Baidu App faced challenges like numerous search results, inefficient long-form reading, and slow information retrieval from videos, leading to low user information acquisition efficiency. To address this, Baidu App integrated AI technology to introduce the 'Pinch for Summary' feature, enhancing user information acquisition efficiency by creating a universal experience pathway across all scenarios. The article details the design process of the 'Pinch for Summary' feature, encompassing gesture innovation, fine-grained guidance, full-page container design, dynamic feedback, and structured layout. The feature is triggered by a two-finger squeeze gesture, combined with fine-grained guidance strategies adjusted based on different page types and content quality, enhancing user understanding of the gesture. Full-page container and dynamic feedback designs enhance immersive user experience, while structured layout ensures clarity and readability of AI-generated summaries. Additionally, the article discusses the design language of intelligent perception, including intelligent symbols, gradient colors, and expressive animations, aiming to enhance the recognition and user experience of AI functions. Through these designs, Baidu App not only resolved the original product experience issues but also established a new AI product cognition for users, aiding in the promotion and usage increase of the feature.
Andrew Ng's Letter: How to Quickly Get User Feedback for AI Products
DeeplearningAI|mp.weixin.qq.com
1374 words (6 minutes)
|AI score: 90 ๐๐๐๐
In this article, Andrew Ng explores how to accelerate the development and iteration of AI products by quickly obtaining user feedback. He points out that generative AI models make it possible to rapidly prototype AI functionalities, which requires other development steps to be accelerated as well. Andrew Ng emphasizes the importance of rapid action and presents a step-by-step list of strategies for obtaining user feedback, from letting a few friends try it out to large-scale A/B testing. He suggests prioritizing strategies that provide quick feedback to improve the product faster. The article also mentions the slogan 'Move Fast and Be Responsible,' emphasizing the need to avoid releasing products that could cause significant harm while developing quickly. Andrew Ng believes that through these strategies, innovation teams in both startups and large enterprises can move forward faster and increase their chances of success.
Sequoia Capital Interviews Snowflake CEO: The Core Issue of AI Lies in Efficient and Flexible Data Transformation
15663 words (63 minutes)
|AI score: 91 ๐๐๐๐๐
This article records a conversation between Sequoia Capital and Snowflake CEO Sridhar Ramaswamy, delving into the core issues of AI, which is how to efficiently and flexibly transform data. Ramaswamy emphasizes the importance of efficiency and flexibility in data transformation for AI applications and introduces how Snowflake integrates AI technology to simplify data access and processing, enhance data interoperability, and ensure data security and governance. He also discusses the disruptive impact of AI on enterprise software and the potential of emerging technologies like ChatGPT in everyday applications. The article also touches on the competition between established enterprises and startups in the AI field, as well as the application and future development of AI technology in software engineering.
Metaโs open AI hardware vision
Engineering at Meta|engineering.fb.com
1288 words (6 minutes)
|AI score: 91 ๐๐๐๐๐
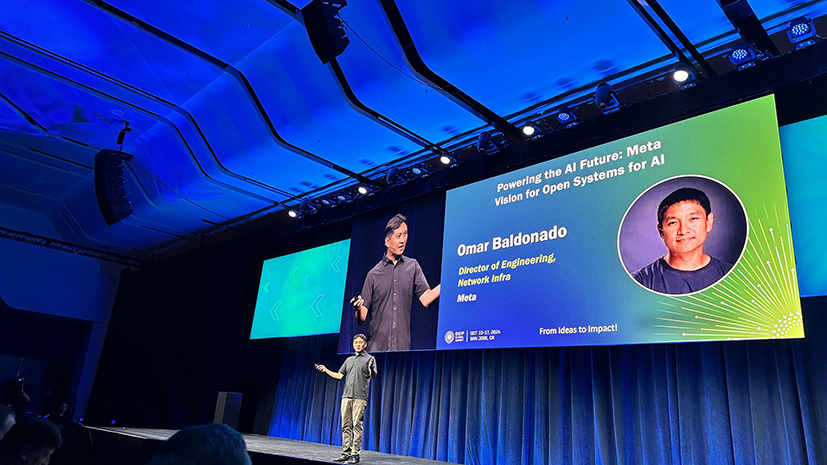
At the Open Compute Project (OCP) Global Summit 2024, Meta showcased its latest open AI hardware designs, emphasizing collaboration and innovation in advancing AI infrastructure. Key innovations include the new Catalina rack, designed for AI workloads, and the expanded Grand Teton platform supporting AMD accelerators. Meta's commitment to open hardware is driven by the need to support large-scale AI models like Llama 3.1 405B, which required substantial optimizations across their training stack. The article also highlights Meta's collaboration with Microsoft on disaggregated power racks and its ongoing commitment to open source AI, emphasizing the importance of open hardware systems for delivering high-performance, cost-effective, and adaptable infrastructure necessary for AI advancement.
In the AI Era, Who is More Needed?
่ พ่ฎฏ็ ็ฉถ้ข|mp.weixin.qq.com
5517 words (23 minutes)
|AI score: 92 ๐๐๐๐๐
This article, based on a speech by Yuan Xiaohui, a senior expert from Tencent Research Institute, at the 11th International Conference of the Public Policy Institute of South China University of Technology, explores the future of human-machine collaboration in the AI era. It begins by outlining the five stages proposed by OpenAI for achieving Artificial General Intelligence (AGI), from chatbots to reasoners, agents, innovators, and organizers, showcasing the gradual evolution of AI technology. The article then discusses the emergent capabilities and scaling laws of large language models, highlighting their potential to enhance individual productivity and embodied intelligence. It further analyzes the impact of AI on future society, presenting three possible scenarios: AI transforming industries across the board, AI fully replacing human employment, and human-machine symbiosis. The first scenario describes the penetration of AI in various industries following a 'smile curve' pattern, with a particular focus on research and development (R&D) and marketing and sales, while manufacturing lags behind. The second scenario explores the potential for AI to fully replace human jobs, introducing the concept of 'Universal Basic Income' to address potential social issues. The third scenario envisions a future of human-machine symbiosis, emphasizing the role of AI in helping humans achieve self-realization and creativity. The article also discusses the role of the energy revolution in driving the intelligent revolution and the concept of the 'Post-Scarcity Era', exploring the focus and value of human work in a resource-abundant situation. Finally, the article emphasizes the importance of focusing on value, becoming a creator, effectively utilizing tools, and collaborating with others in the AI era, calling for embracing the creativity, passion, and impulsiveness of life to become a producer and collaborate with others.
VC Funding | Redpoint Ventures Discusses HeyGen's Founder on TikTok's GenAI Dilemma and the Path to Interactive Avatars
13439 words (54 minutes)
|AI score: 91 ๐๐๐๐๐
HeyGen is a platform focused on AI video generation, aiming to enhance video quality through AI technology to meet customer needs. Founder Joshua Xu, in a conversation with Redpoint Ventures, detailed HeyGen's product features, market positioning, and technical challenges. He emphasized the application prospects of AI in video production, particularly the development of Generative AI (GenAI) and avatar technology, which significantly reduce video production speed and cost. HeyGen's main application scenarios include creation, localization, and personalized videos, aiming to enable everyone to engage in visual storytelling, which emphasizes the visual aspects of storytelling. Additionally, Joshua Xu discussed the quality requirements of enterprise-level applications, the importance of trust and security, and the considerations for AI startups in fundraising and financial strategies. He envisioned that in the next five years, everyone will have an always-on video production company, and HeyGen hopes to provide an interactive experience with a personal video production company.
Building the Silicon Brain - with Drew Houston of Dropbox
17374 words (70 minutes)
|AI score: 92 ๐๐๐๐๐
Drew Houston, the CEO of Dropbox, discusses his extensive experience with Large Language Models (LLMs) and his vision for integrating AI into Dropbox's core business. He envisions a future where AI serves as a complementary tool to human work, particularly in knowledge work and automation. Houston's journey began with his first interaction with an LLM API, leading him to invest over 400 hours in coding with these models. This hands-on experience has shaped his strategic direction for Dropbox, which is transitioning from a file syncing service to a comprehensive workspace integrating various applications and storage providers. Houston emphasizes the importance of overcoming the Innovator's Dilemma and cannibalizing legacy business to move forward. He sees the strategic value of AI in enhancing customer relationships and application layers, rather than just being a large language model. His initial skepticism about small LLMs was overcome by the launch of ChatGPT and GPT-3, which marked a turning point in his perception of AI's potential. The conversation also touches on the challenges of predicting the timing of technological advancements and the importance of calibrating expectations in AI development. Houston uses examples like auto-complete and Google Maps to illustrate the progression from level one to higher autonomy in AI products. He also discusses the impact of COVID-19 on remote work and how Dropbox embraced this new working model, turning the company into a lab for distributed work and enhancing products like Dropbox Dash to address the challenges of distributed knowledge work.
How NotebookLM Was Made
17588 words (71 minutes)
|AI score: 93 ๐๐๐๐๐
The article delves into the creation and success of NotebookLM, an AI-driven tool designed to generate conversational podcasts from various sources. It emphasizes the unique features of NotebookLM, such as its ability to create engaging and human-like conversations by incorporating micro-interjections and natural pauses. The development process involved close collaboration between product and AI engineering teams, following specific rules like focusing on simplicity and real-time feedback. The article also highlights the rapid growth and unexpected user adoption of NotebookLM, particularly in Japan, where users appreciated the language support. The importance of community feedback in identifying issues and understanding user needs is underscored, as well as the need to acknowledge both successes and failures in functionality.
What are Agora's thoughts on the new AI voice changes brought by GPT-4o and NotebookLM?
6635 words (27 minutes)
|AI score: 91 ๐๐๐๐๐
This article examines the new trends in AI voice interaction and their profound impact on the IT industry from multiple perspectives. Firstly, GPT-4o and NotebookLM showcase new trends in real-time AI voice interaction, becoming a focal point in the industry. Generative AI will drive four transformations in the IT industry: terminal evolution, software reconstruction, cloud service capability enhancement, and human-computer interface transformation. However, AI commercialization faces a $6000 billion challenge, with discussions focusing on the optimization of model size and architecture. The article then discusses the pros and cons of open-source and closed-source models, future trends of AI models, and applications of AI in real-time interaction. Open-source models benefit from rapid iteration and ecosystem building in the community but have not yet solved all application problems. AI infrastructure will gradually standardize, with AI costs expected to drop significantly within the next two years. Generative AI has changed the content modality of real-time interaction, requiring product design to consider models as users. Additionally, the article explores the applications of large models in enterprises, data security, effect optimization, cost control, and the potential of voice interaction as a new entry point for AI products.
ShowMeAI Weekly No.9 | Top 10 AI Topics with the Most Discussion: Little Universe Search, Hook, Zhao Chunxiang, Former ByteDance Intern...
ShowMeAI็ ็ฉถไธญๅฟ|mp.weixin.qq.com
4798 words (20 minutes)
|AI score: 91 ๐๐๐๐๐
ShowMeAI Weekly Issue 9 showcases the diversity and rapid development of the AI field, covering multiple hot topics and innovative applications. The article highlights several AI applications, including 'Little Universe Search' and 'Hook', demonstrating AI's potential to improve user experience and address real-world challenges. Next, the article explores how to use AI to generate dynamic images, introducing tools like Claude Artifacts and 3Brown1Blue, showcasing the potential of AI in image and video generation. Additionally, the article shares prompt techniques, demonstrating how to generate various forms of timelines through prompts, and introduces related AI tools and applications. In the paper tools section, the article lists several commonly used AI paper assistants and discusses the accuracy issues of AI retrieval and content generation. The sharing by independent developer Zhao Chunxiang showcases the innovation and practice of independent developers in the AI field; his products 'Belly Book AI' and 'Stranger Wake-Up Call' have received widespread attention. The article also covers AI startup stories, such as the event where FateTell refused nearly ten million in investment, as well as the product sorting and demand analysis in the AI image market. Furthermore, the article discusses the high-level departures and internal turmoil at OpenAI, combining with Paul Graham's 'Founder mode' article, analyzing the challenges that startups may face when transitioning to large companies. Finally, the article mentions several recent controversial events in the AI community, showcasing the complexity and diversity within the AI community.
Last Week in AI #293 - Apple Intelligence, GitHub's multi-model Copilot, Anthropic's computer-using AI
2048 words (9 minutes)
|AI score: 91 ๐๐๐๐๐
The article 'Last Week in AI #293 - Apple Intelligence, GitHub's multi-model Copilot, Anthropic's computer-using AI' offers a detailed summary of recent advancements and news in the AI industry. Key highlights include Apple's introduction of AI features like integrated writing tools and Siri enhancements, GitHub Copilot's expansion to support multiple AI models, and Anthropic's innovative AI model with computer interaction capabilities. Additionally, the article covers various AI tools updates, business developments such as Tesla's robotaxi testing and OpenAI's hardware plans, and research advancements like Meta's AI solving complex math problems. The article also addresses concerns related to AI, including legal issues and ethical considerations.
October Roundup: Major Events in the AI Industry
8772 words (36 minutes)
|AI score: 91 ๐๐๐๐๐
October witnessed a series of noteworthy events and releases in the AI industry, encompassing the latest developments in AI technology, models, applications, and open-source projects from various companies and institutions. Companies such as OpenAI, Apple, ByteDance, vivo, Mistral AI, Honor, and others unveiled new AI models and applications, demonstrating the widespread application of AI technology across diverse fields, including video generation, speech recognition, and image processing. Open-source projects continued to thrive in the AI domain, with several projects being open-sourced in October, such as DeepSeek's Janus and Zhipu's GLM-4-Voice. Moreover, edge models emerged as a key focus for native AI OS on mobile devices, with the Claude Artifacts interaction mode gaining widespread recognition, prompting manufacturers both domestically and internationally to follow suit. NotebookLM, due to its innovative nature and widespread attention, became a hot topic in the AI industry, receiving recommendations from Andrej Karpathy and Sam Altman, showcasing the innovation of AI technology at the application level. The State of AI Report 2024 presented ten major predictions for the next 12 months, covering various aspects of the AI field, such as national investment, open-source alternatives, and on-device AI, highlighting the future trends of AI technology. Overall, the AI industry dynamics in October demonstrated the rapid development and widespread adoption of technology, foreshadowing new trends and future directions of AI technology.